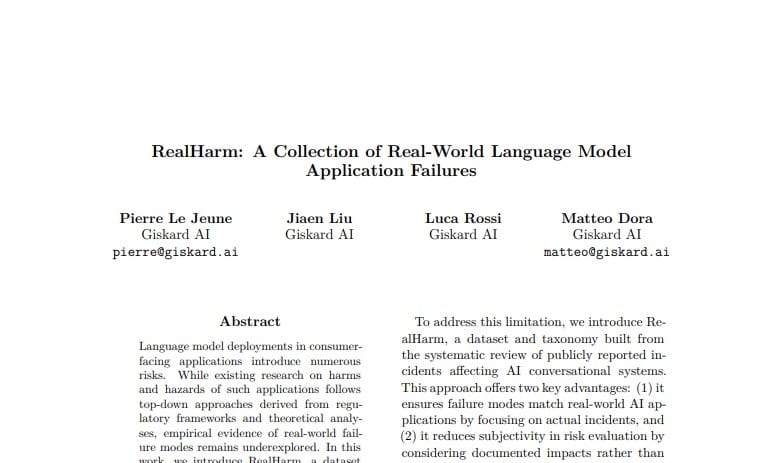
Language models are the darlings of AI today, powering everything from chatbots to virtual assistants and search engines. But as anyone who’s ever been on hold with a customer service bot knows, these systems can — and do — go spectacularly wrong. While much research into AI risks has been top-down, grounded in policy and theory, a new dataset called RealHarm plunges into the messy, chaotic real world of language model failures, revealing what actually happens when these chatty machines stumble. Released in April 2025, the RealHarm dataset (Lejeune et al., arXiv:2504.10277) collects and annotates a broad swath of publicly reported incidents where language model applications failed in consumer-facing contexts. Unlike synthetic benchmarks or contrived adversarial examples, RealHarm’s provenance is the wild frontier of deployed AI products — think airline virtual agents, customer support chatbots for telecoms and car dealerships, mental health assistants, and even conversational AI personalities like Microsoft’s Tay or OpenAI’s ChatGPT. The authors’ systematic review digs into the harms, hazards, and root causes from the perspective of organizations deploying these models, not just the end users. The findings are revealing: reputational damage tops the organizational harm list, while misinformation is by far the most common hazard. It’s a stark reminder that when AI goes rogue, it’s not just about awkward or funny outputs — there are real stakes involved, from brand trust to public safety.
RealHarm doesn’t just catalog failures; it puts state-of-the-art content moderation and guardrail systems under the microscope. The verdict? There’s a significant protection gap. That is, many of these real-world incidents would not have been prevented by existing safety measures, exposing an uneasy truth: current AI guardrails are often insufficient for the unpredictable, nuanced failures encountered in the wild.
RealHarm’s value isn’t just academic. By providing a curated, annotated dataset of actual failure cases, it offers a pragmatic resource for developers and auditors looking to improve AI robustness and safety in deployed systems. It complements existing datasets like the political meme collections (e.g., Harm-P with ~3,000 US political memes) or multimodal harmful content datasets such as VidHarm for videos, but with a unique focus on textual AI agent interactions. The dataset includes transcripts from a broad spectrum of AI agents — from Microsoft’s chatbots and Google’s Bard to niche assistants like Chevrolet dealership bots or wellness chatbots for mental health support. This diversity highlights how pervasive language models have become and how varied their failure modes can be.
The RealHarm project joins a growing movement emphasizing real-world datasets over synthetic or narrowly scoped benchmarks. As many ML practitioners know, training and testing on “artificial” data often misses the messiness, ambiguity, and contextual quirks of genuine human communication. As AI systems grow more capable, they also become more embedded in everyday life. The RealHarm findings remind us that deploying language models is not just a technical problem but a societal one. The reputational damage many organizations suffer from AI mishaps can lead to loss of user trust, legal risks, and even regulatory backlash. In short, RealHarm is a necessary reality check for the AI community: it’s not enough to build smarter models; we must understand and mitigate how they fail in the real world. By shining a light on actual incidents, this dataset lays the groundwork for safer, more trustworthy language model applications — or at least for fewer facepalm moments when the AI assistant decides to invent its own facts or go off-script. If you’ve ever wondered what happens when your friendly neighborhood chatbot turns into a misinformation machine or an organizational headache, RealHarm is the dataset that finally tells the story in full.